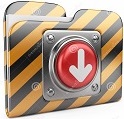
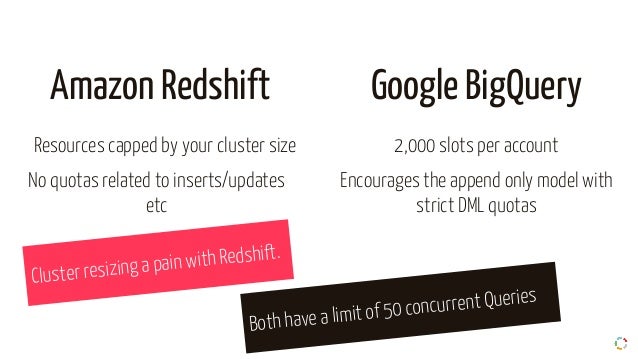
👇🏼 The data engineer's role today encompasses the entire data engineering process, requiring familiarity with the data engineering lifecycle and evaluating data tools for optimal performance across various dimensions. 👇🏼 Concepts like the semantic layer help maintain a common understanding of data across systems. 👇🏼 #Python and #SQL are the essential languages for data engineers. 👇🏼 The shift towards data products and the data mesh architecture emphasizes the idea of data as a product. 👇🏼 Concepts like functional data engineering, idempotency, and declarative programming have been adopted in data engineering for better pipeline operability and observability.
Bigquery vs redshift software#
👇🏼 Data engineers adopt software engineering best practices, including agile development, code testing, and version control.

👇🏼 Contemporary data engineers focus on higher-value tasks such as data modeling, quality, security, management, architecture, and orchestration due to data tools' abstraction of Big Data frameworks. 👈🏼 The title of "big data engineer" was mostly replaced by the broader term "data engineer." 👈🏼 The modern data stack emerged in the early 2010s, with cloud data warehouses like #Redshift, #BigQuery, and #Snowflake, along with tools for orchestration (Airflow), transformation (dbt), and BI (Looker, Metabase, Mode, etc.). 👈🏼 The advent of public cloud providers (AWS, Google Cloud, Microsoft Azure) revolutionized software and data applications, allowing companies to save on up-front costs and easily scale resources. 👈🏼 The early 2000s saw the rise of Big Data, driven by tech giants like Google, Yahoo, and Amazon, and the development of the Hadoop framework. 👈🏼 Data warehousing started in the 1980s, leading to scalable analytics through MPP databases. A concise exploration of #dataengineering: 18 bullet points unveiling its past, present, and future ✨
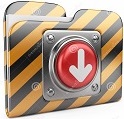